Σημασιολογική Ομοιότητα Κειμένων - Notion Oriented Approach (NOA)
Text Semantinc Similarity - Notion Oriented Approach (NOA)
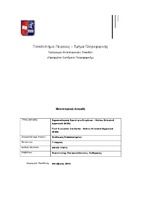
View/ Open
Abstract
Natural Language Processing is a field of Artificial Intelligence that accompanies Computer Science through it's early steps. It bifurcates into various branches with most important these of translation, spelling errors correction that most modern text processors use and speech recognition or Text-To-Speech algorithms. Even though these are the most known to the public categories, the community of Artificial Intelligence that works on Natural Language Processing, has a huge variety of sub-domains to choose, which also includes Named Entity Recognition, Summary Extraction, Auto Question Answering, Text Semantic Similarity, etc. Text Semantic Similarity is the one that this dissertation deals with and probably is one of the most complex problems that researchers of NLP tries to solve. Even if the first models were created enough years ago, the various approaches change periodically, in a journey of seeking for the most robust and feasibly, optimized solution in the current problem.
The current approaches, have proposed models based on metrics of two basic categories, Knowledge-Based and Corpus-Based metrics. Those metrics have been used solely or combined in various approaches that deal with the Text Semantic Similarity problem. Up to now, none of the proposed models can answer with total reliability and confidence if two texts are semantically identical. Most models return a normalized value that defines the percentage of similarity between input texts and this techniques are used
mainly for text categorization or clustering, depending on their subject. Best systems evaluation results up to 85% of texts, which is a good percentage, but not adequate for applications that will produce reliable models based in natural language.
The current dissertation deals with this problem, proposing a new Knowledge-Based model, which, unlike the majority of other models that use WordNet or Wikipedia as a Knowledge Base, proposes a new Knowledge Base, which covers in depth its functionality. This Knowledge Base is based on the work of Collins & Quillian, and represents the words and the notions that are related to, as a semantic network. With the Knowledge Base described above and the application of rules, based on Hidden Markov Models (HMMs) the proposed model can result a boolean value that will define if the two input texts are semantically similar or not, with respect in grammatical and syntactical rules of the language that has been used for the examined texts, which play a huge role in further disambiguation of the semantic meaning of a sentence.