Μερικά παρατηρήσιμες μαρκοβιανές διαδικασίες αποφάσεων και εφαρμογές σε προβλήματα αντικατάστασης συστημάτων και επιλογής διδακτικών μεθόδων
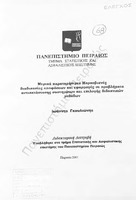
View/ Open
Subject
Μαρκοβιανές ανελίξεις ; Διαχείριση κινδύνου -- Οικονομετρικά μοντέλα ; Διαχείριση κινδύνου -- Στατιστικές μέθοδοι ; Risk management -- Econometric models ; Risk management -- Statistical methods ; Markov processes ; Teaching -- Methods ; Διδασκαλία -- Μεθοδολογία ; Εκπαίδευση -- Στατιστική ; Educational statisticsAbstract
A Partially Observable Markov Decision Process (POMDP) is a natural extension of the Markov Decision process (MDP).In POMDPs the state of the system is not observable and therefore unknown Instead, the decision maker receives a random signal that depends on the state of the system at the beginning of each epoch and then he chooses an action from a finite set of actions. Starting with an initial prior information vector, belief state, (i.e. a probability distribution on the state space), it is updated at beginning of each time epoch just after the arrival of a signal. The new information vector (or belief state) is the posterior distribution on the state space using Bayes' rule that involves the transition and observation matrices assigned to the action selected at the previous time epoch. It is well known that information vector incorporates the information of the history of the system when choosing an action at a time epoch. The immediate costs (rewards) depend on the current state and action. The objective is the calculation of the optimal expected total discounted cost (reward) with respect to finite or infinite horizon and the determination of the optimal policy. Although POMDP may provide as suitable model for many applications they may be severely limited due to the computational complexity. Within this context the main goals of this thesis are as follows. Firstly, the development of flexible algorithms for the determination on optimal or near optimal policies, as well as approximations of the optimal reward or (cost) functions for finite or infinite horizon. Secondly, to find alternative conditions or generalize known conditions that ensure that a given stationary policy induces a Markovian partition of the belief state space. In this case the reward (or cost) function for infinite horizon is piecewise linear function and its evaluation is significantly simplified. Thirdly, application of the POMDP model in problems of repair / replacement of the system. It is assumed that the system is monitored incompletely by a certain mechanism which gives the decision maker some information about the exact state of the system. Fourthly, modeling a teaching methods selection problems as POMDP, where the state of the class (the degree of comprehension teaching material) is unknown to the teacher, and instead signals of success / failure type in tests are received.