Ανίχνευση μορφολογικών χαρακτηριστικών βλαστοκύστεων με χρήση μοντέλων σημασιολογικής τμηματοποίησης
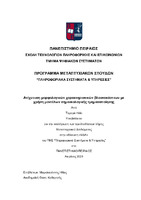
View/ Open
Keywords
Ανάλυση βλαστοκύστεων ; Εξωσωματική γονιμοποίηση (IVF) ; Εσωτερική κυτταρική μάζα (ICM) ; Τροφεκτοδερμία (ΤΕ) ; Πυκνή μάζα (ZP) ; Τμηματοποίηση ; Σημασιολογική τμηματοποίηση ; UNet ; DeepLab ; PSPNetAbstract
The aspiration of this thesis is the development and comparison of three seman-tic segmentation models, UNet, DeepLab, and PSPNet, for the detection of three mor-phological characteristics (ICM, TE, and ZP) from blastocyst images, aiming to assess embryo suitability for in vitro fertilization (IVF). These models exhibit different behaviors with respect to the dataset used for training and evaluation.
This thesis highlights the significance of image segmentation in machine learning, particularly in the medical field, as well as the three main categories of segmentation, along with relevant application examples. It explores artificial neural networks (ANNs) and their various types, such as feed-forward neural networks (FFNs), convolutional neu-ral networks (CNNs), and recurrent neural networks (RNNs), which are widely used in semantic segmentation tasks. However, the primary focus is on the UNet, DeepLab, and PSPNet models, providing a detailed analysis of their architectures, the hyperparameters used for training, and their evaluation methods. Finally, the research methodology is out-lined, followed by a presentation of the obtained results.
This study’s effort to detect the three morphological characteristics (ICM, TE, and ZP) in blastocysts identified the UNet model as the most suitable, as it demonstrated the best overall performance compared to the other two models. The UNet model proved to be the most stable, with strong generalization capabilities and no overfitting issues. The Intersection over Union (IoU) metric evaluation for the detection of ICM is reported to be 67.05%, 56.88% for TE, and 63.65% for ZP. Lastly, suggestions for improvements are provided in order to enhance the performance of the DeepLab and PSPNet models, as well as strengthen the foundation for the future research in the field of semantic segmen-tation of medical images, particularly blastocyst images.