Εφαρμογή τεχνικών μηχανικής μάθησης γράφων για ανίχνευση απάτης συναλλαγών
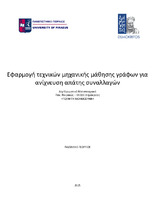
View/ Open
Keywords
Θεωρία γράφων ; Μηχανική μάθηση ; Βαθιά μηχανική μάθηση ; GCN ; GNNAbstract
Fraud detection in financial transactions constitutes a critical issue in the field of finance, as the rapid advancement of technology has increased the frequency and complexity of fraudulent activities. Traditional data analysis methods often prove inadequate in addressing the growing complexity of these problems. In this context, graph machine learning algorithms emerge as an innovative and powerful approach to fraud detection, as they enable the exploitation of structural features and relationships within the data.
This thesis examines the application of graph machine learning algorithms in detecting fraud in financial transactions. Modern techniques such as Graph Neural Networks (GNNs) and Graph Convolutional Networks (GCNs) are analyzed, while their advantages and limitations are explored in real-world scenarios. Through the integration of features such as graph topology, correlation analysis, and data dynamics, the thesis presents a framework aimed at improving the accuracy and effectiveness of fraudulent transaction detection.
The research findings suggest that graph machine learning algorithms can reveal hidden fraud patterns, offering a powerful tool to combat fraud in financial transactions. The study concludes with recommendations for future research and potential applications in industrial settings.