Σχεδιασμός, υλοποίηση και δοκιμή τεχνικών μηχανικής μάθησης για την ανίχνευση κυβερνοεπιθέσεων
Design, implementation and testing of machine learning techniques for cyberattack detection
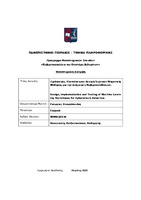
View/ Open
Keywords
Μηχανική μάθηση ; Κακόβουλο λογισμικό ; Ταξινόμηση ; MLP ; SVC ; CatBoost ; Borda ; Malimg ; Συνελικτικά νευρωνικά δίκτυα ; Στρωματοποιημένη Διασταυρούμενη Επικύρωση K-Fold ; ResNet-50 ; DenseNet-121 ; Bayes ; SMOTE ; ANOVAAbstract
Cyberattack detection using Machine Learning has emerged as one of the most dynamic fields of
research, enabling the real-time analysis of large datasets and the identification of abnormal be
haviors with significantly greater accuracy compared to traditional methods. This study focuses
on the classification of malware represented images, into five distinct categories: Backdoors &
RATs, Downloaders & Droppers, Multipurpose, Spyware & Adware, Rogue Software & Fraud
ware, and Worms & Self-Replicating Malware. A Transfer Learning approach was adopted, utiliz
ing features extracted from the publicly available Malimg dataset through the integration of two
Pre-Trained on ImageNet deep learning architectures — ResNet-50 and DenseNet-121. Bayes
ian Optimization was employed to determine the optimal number of layers to unfreeze for fine
tuning, enhancing the quality of feature extraction. Subsequently, feature selection was performed
using the ANOVA method. For classification, three model families were explored: a non-linear
model (Support Vector Classification – SVC), a neural network (Multi-Layer Perceptron – MLP),
and a tree-based ensemble model (CatBoost). All models underwent hyperparameter optimiza
tion via Bayesian search. Results demonstrated exceptional performance, with all evaluation met
rics — including per-class (accuracy, precision, recall, F1-score, specificity, ROC AUC), averaged
(macro, weighted), and global (micro metrics, balanced accuracy, Cohen’s Kappa, Matthews Cor
relation Coefficient) consistently exceeding 98%, and in most cases, 99%. Finally, model combi
nation using the Borda count method further improved accuracy, yielding only 35 misclassifica
tions out of a total of 8,024 samples.