Πολυγλωσσικοί ταξινομητές μέσω νευρωνικής μηχανικής μετάφρασης με έμφαση σε μεθόδους εκμάθησης μέσω μεταφοράς και ομοσπονδιακής μάθησης
Multilingual classifiers based on neural machine translation with an emphasis on transfer and federated learning methods
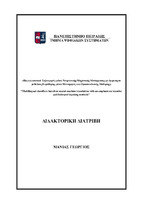
View/ Open
Keywords
Πολυγλωσσικοί κατηγοριοποιητές ; Πολυγλωσσική ταξινόμηση κειμένου ; Εκμάθηση μέσω μεταφοράς ; Επεξεργασία φυσικής γλώσσας ; Ανάλυση συναισθήματος ; Ομοσπονδιακή μάθηση ; Νευρωνική μηχανική μετάφρασηAbstract
In recent years, there has been a remarkable increase in the interest of the scientific and business
communities in the field of Artificial Intelligence – AI. The ability of modern devices to
implement highly sophisticated AI techniques has increased the importance of using and
developing applications that will use AI techniques and algorithms to process and analyze
modern data. Undoubtedly, progress in AI makes computer systems more human-friendly, which
requires the inclusion of natural language as an integral means of broader communication and
interface between humans and computers. Today, the primary goal of research in the field of
Natural Language Processing – NLP is to enable and facilitate communication between humans
and computers without resorting to complex procedures. In particular, the introduction into our
daily lives of a large number of applications that fall within the field of Generative Artificial
Intelligence – GAI, such as ChatGPT by OpenAI, Copilot by Microsoft, and Google Bard, have
introduced revolutionary methods in the fields of NLP, Natural Language Understanding – NLU,
and Natural Language Generation – NLG of more human-friendly linguistic content. The latter
has also brought significant improvements in human-machine interaction, allowing for faster
production of text and other audiovisual material. Modern chatbots, automatic translation
machines, and search engines are just some of these applications. These applications apply
algorithms and techniques that fall within the field of AI, which is a subfield of AI and is one of
the most advanced and growing research and business areas of the last five years.
Although AI is not a new field of research, recent technological developments in the context of
computer science, the increasing need for meaningful human-computer communication, the
availability of big data, high-power computing resources and modern algorithms have triggered
an exponential increase in interest in the development of new applications and systems. However,
one of the biggest challenges in AI is the inability to generalize systems so that they can be
effectively applied to multiple and heterogeneous areas of interest. Emerging Machine Learning
– ML techniques and approaches, such as Transfer Learning, aim to address these limitations.
However, the ever-increasing production and diversity of data create additional challenges in
interoperability and their use in different sectors. Moreover, one of the major problems faced by
modern applications in the field of NLP has to do with their inability to generalize and apply to
different areas of interest. The modern production of huge volumes of data and the diversity that
distinguishes them places certain limitations on their application and interoperability between
different sectors, such as health, public administration, industry, etc. Sentiment analysis or entity
recognition applications that have been created for a specific sector, e.g. in the healthcare sector,
appear to have lower performance if used in a different sector, e.g. sentiment analysis of social
media users regarding an electoral process or a specific product. This demonstrates the need to
develop techniques that are not limited by the content and domain in which they are developed,
but are based on the concepts of interoperability and data federation.
Of particular importance is the need to develop systems that support multilingual
implementations. Language differences constitute a significant obstacle to the horizontal
application of NLP algorithms, as most implementations focus on specific languages, resulting
in limited application and performance in different linguistic or cultural contexts. The
implementation of multilingual systems is a critical requirement for the creation of interoperable
and federated approaches that do not depend on language, thus ensuring their wider use and
integration. The need for multilingual implementations is not limited only to addressing language
barriers but also contributes to expanding the usefulness of systems at a global level, enhancing
accessibility, inclusiveness and equitable development. Systems with multilingual support
enhance the ability to manage data from distributed and heterogeneous sources, unifying
knowledge and blurring language boundaries, thus offering more accuracy and flexibility in
different application areas.
ΠΕΡΙΛΗΨΗ
ΜΑΝΙΑΣ ΓΕΩΡΓΙΟΣ – ΔΙΔΑΚΤΟΡΙΚΗ ΔΙΑΤΡΙΒΗ 18
Furthermore, the production and management of big data from more and more distributed
sources and devices creates the need to develop federated and interoperable approaches that are
not influenced and limited by a single language. In recent years, the use of Federated Learning,
where devices are used as computing nodes that perform calculations on their local data in order
to update a global model, aims to solve the issue of data federation, while simultaneously
enhancing the security of user data. Finally, the needs and trends of modern intercultural and
multilingual societies increasingly demonstrate the need to create multilingual solutions and
applications that will have the ability to operate in a broader federal context and will make full
and effective use of Neural Machine Translation – NMT techniques. So far, the research and
business community have focused on the application of tools and techniques based on the English
language. Few studies and tools target less important languages, such as Greek. For example,
despite the continuous advances in the development of NMT algorithms and techniques for
sentiment analysis in high-frequency and high-use languages, such as English, few applications
and studies have been carried out in less used and more difficult languages, such as Greek.
Based on the above challenges, this doctoral thesis focuses on the development of scientific
approaches that will promote multilingual interoperability and generalization of AI applications.
The goal is to create innovative systems that can manage data from heterogeneous and distributed
sources, ensuring effective interaction between humans and machines regardless of language or
cultural context, and enhance the decision-making process by providing reliable results and
additional knowledge to end users. Through the integration of techniques such as transfer
learning, pre-trained language models, federated learning and NMT, the thesis aspires to
contribute to addressing contemporary research and technological limitations, laying the
foundation for solutions that will enhance fair and inclusive development in the field of AI.