Investigating the generalization abilities of bottom-up visual saliency models in task-specific scenarios using transfer learning
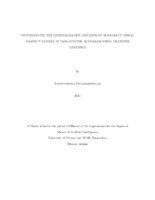
Master Thesis
Author
Chaldaiopoulos, Konstantinos
Χαλδαιόπουλος, Κωνσταντίνος
Date
2024View/ Open
Keywords
Visual saliency ; Saliency prediction ; Deep learning ; Transfer learningAbstract
In this thesis, we combine knowledge from two different topics: the first is computational models of attention and free-viewing fixation prediction, and the second is transfer learning and the generalization ability of deep learning models.
Inspired by human transfer learning abilities, we hypothesized that a fixation model trained on fixation maps representing human free-viewing behavior would have increased generalization abilities compared to a task-optimized model.
To test this hypothesis, we created different experimental scenarios that aimed to extensively compare the generalization abilities of these two models or agents. In these scenarios, we introduced two animal image segmentation tasks between the agents: one similar and one dissimilar to that of the task-optimized agent.
We concluded that, for our experimental conditions, the fixation agent did exhibit increased generalization abilities, both when direct training was applied to each task separately and when retraining was performed successively.