Σύγκριση αλγορίθμων μηχανικής μάθησης στην εκτίμηση πιστωτικού κινδύνου
Comparison of machine learning algorithms in credit risk assessment
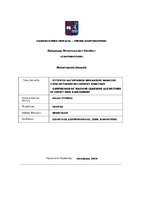
View/ Open
Keywords
μηχανική μάθηση ; πιστωτικός κίνδυνος ; ανισορροπία κατηγοριώνAbstract
Credit risk assessment is a critical issue for financial institutions, as an accurate
assessment of the probability of default on a loan is essential to avoid financial losses. In
this study, credit risk assessment is treated as a binary classification problem between the
categories of (a) defaulting and (b) non-defaulting borrowers. The dataset "Home Credit
Default Risk" which contains real data from the Kaggle platform is used to train and compare
the performance of the machine learning models, Logistic Regression, Random Forest,
XGBoost and LightGBM, in order to assess the creditworthiness of borrowers. This was
preceded by exploratory data analysis and data preprocessing as well as feature generation
to enrich the data and improve the performance of the models. In addition, feature selection
techniques were used based on the feature importance through the use of LightGBM, and
the application of PCA to reduce the dimensionality of the features was also considered.
In the dataset there was a large class imbalance between the two categories, with the
majority of borrowers not defaulting, which led to the testing of balancing techniques such
as SMOTE and SMOTEENN to improve the models' ability to identify those who default. For
the validation of the results we used the Stratified KFold method while the performance of
the models was evaluated using the Confusion Matrix and other metrics as the ROC-AUC,
F1-Score, Precision and Recall, with the LightGBM model proving to be the most efficient in
the majority of the tests achieving prediction accuracy (ROC-AUC=0. 7865) and detecting
instances of default (Recall=0.67). However, although LightGBM performed better
compared to the other models, the overall results remain unsatisfactory as the models are
not able to adequately identify the categories due to the strong imbalance. This difficulty
highlights the need to explore more specialized balancing techniques and the use of
machine learning methods capable of better addressing class imbalance problems. The
adoption of automatic feature generation techniques and optimization of model
hyperparameters could lead to significant improvements in model performance, resulting
in improved forecasting and better credit risk management.