Ενισχυτική μάθηση - Ms Pacman
Reinforcement learning - Ms Pacman
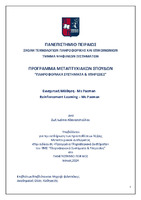
View/ Open
Keywords
Ενισχυτική μάθηση ; Deep Q-Network ; Batch normalization ; Duel DQN ; Noisy DQN ; Prioritized experience buffer ; Ηλεκτρονικά παιχνίδια ; Εκπαίδευση ; Google Colab ; GPU ; MsPacman ; Python ; PyTorch ; Παιχνίδι Arcade ; Πράκτορας ; AzureAbstract
This thesis explores the application of reinforcement learning for training agents in video games. The
purpose of the research is to investigate the performance and effectiveness of various Deep Q-Net
work (DQN) algorithm variations in arcade game environments.
Initially, the thesis presents the basic concepts of reinforcement learning, including policies, reward
functions, value functions, and environment models. Then, it analyzes the theoretical principles of
DQN algorithms and their extensions, such as DQN with batch normalization, Duel DQN, Noisy DQN,
and Double DQN with Prioritized Experience Buffer.
Experimental results show that each variation offers specific advantages in agent training. Agents
trained with the DQN variations showed improvements in average reward, score, and training speed
compared to the basic DQN algorithm. Specifically, the agent with Prioritized Experience Buffer and
Double DQN demonstrated the best overall performance, achieving the highest average reward and
score.
The thesis concludes that reinforcement learning and the adaptations of DQN algorithms can be ef
fectively applied to train agents in dynamic and complex environments, such as video games. The
theoretical analysis and experimental results highlight the significant contribution of optimization
techniques in improving the efficiency and performance of the agents.