Εφαρμογές στατιστικής μηχανικής μάθησης στο μηχανοκίνητο αθλητισμό
Applications of machine learning methods in motorsports
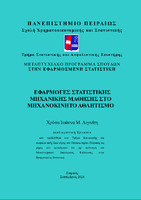
View/ Open
Keywords
Motorsports ; Μηχανοκίνητος αθλητισμός ; Formula One ; Machine learning ; Μηχανική μάθηση ; Regression methods ; Neural networks ; Tree-based methods ; Outcome predictionAbstract
Formula 1 is a domain inextricably linked with speed from the very beginning for a variety of reasons. Speed is embodied in the way the cars race, with drivers constantly testing the limits of both the vehicle and themselves. It is also seen in the rapid emergence and application of innovative ideas that enhance the performance of the car while simultaneously making it exceptionally safe. These innovations are now used in non-racing cars, making everyday transportation both easy and safe. The rapid developments have generated a wealth of data that teams are tasked with processing and interpreting in order to extract hidden information and improve both the car and the strategy they will follow, aiming for victory. To fully and effectively utilize the data, the use of mathematical and statistical methods, as well as computer science, is essential. As a result, the use of statistical machine learning, which successfully combines these three scientific fields, has become inevitable and crucially important for making strategic decisions in fractions of a second during the race, predicting outcomes, and drawing conclusions. This thesis presents an extensive analysis of the various categories that characterize the field of machine learning, the algorithms and models used, and the key methods of data preprocessing before analysis. Finally, statistical machine learning methods are applied to data from F1 races with the aim of predicting the drivers' finishing positions in the race.