Multimodal pretraining for music audio
Πολυμεσική προεκπαίδευση για μουσικά τραγούδια
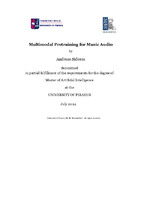
Master Thesis
Author
Sideras, Andreas
Σιδεράς, Ανδρέας
Date
2024-07View/ Open
Keywords
Multimodal ; Audio ; Pretraining ; Music ; Finetuning ; Metric learningAbstract
Data can be expressed in various forms, each potentially encoded through diverse
means. For instance, we might encounter audio data paired with descriptive texts
about their lyrics. Modern systems leverage, if available, the different sources of
information and outperform, under certain conditions, their single-modal
counterparts. In such multimodal settings, each modality encapsulates a distinct
aspect of the underlying semantics of the data and has a supplementary role. Data
can also be limited and without annotations related to the task at hand. In such cases,
transfer learning and pretraining could be two techniques that enhance the
performance of the models. In this thesis, we explore various unsupervised
pretraining techniques while evaluating them on a supervised downstream task. Our
goal is to train a model that can extract meaningful features and be further finetuned
to any new task. We use LLMs to create pseudo-captions that describe the sentiment
and the theme of the lyrics, from a large pool of non-annotated audio. We then
perform a pretraining step, where we learn a multimodal coordinated space between
the audio signals and these pseudo-captions. Then, we finetune our model on an
annotated dataset, where only the audio modality is available. We highlight the ability
of such models to deliver adequate performance in few-shot learning settings, the
incorporation of LLMs into the pretraining step, and the importance of learning a
shared semantic space for information originating from different modalities.