Αξιοποίηση δεδομένων του πραγματικού κόσμου με εφαρμογή τεχνικών μηχανικής μάθησης
Exploiting real world data applying machine learning techniques
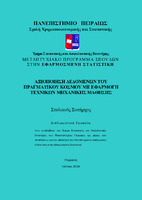
View/ Open
Keywords
Real world data ; Real world evidence ; Machine learning ; Data science ; Biostatistics ; Bionformatics ; FDA ; EMA ; Statistical analysis ; Classification ; Regression ; Diabetes ; Health technology assessment ; Pharmaceutical researchAbstract
In today’s era, data stands as the cornerstone of modern society, encapsulating the essence of the digital landscape and our myriad interactions within it. The collection of data marks a pivotal role in the analysis and extraction of invaluable insights. Today, technological advancements have birthed vast databases across a spectrum of disciplines, among them healthcare, each reservoir of information fostering the growth of knowledge and innovation. Real-World Data and Machine Learning techniques are increasingly pivotal in the field of bioinformatics, revolutionizing healthcare practices and pharmaceutical research and development. This paper delves into the multifaceted landscape of RWD applications, navigating through its distinctions from real-world evidence and its regulatory implications. Exploring the challenges and opportunities presented by RWD, it scrutinizes strategies for harnessing its potential, such as the Observational Medical Outcomes Partnership framework and Health Technology Assessment. Furthermore, it investigates how RWD facilitates drug development through innovative modeling techniques, and how it enables personalized medicine through methods like Social Listening and Quantitative Systems Pharmacology. Additionally, the paper discusses the future trajectory of RWD integration, emphasizing its role in optimizing clinical development and transitioning from data to actionable real-world insights. Presenting insights from library literature in pharmaceutical research, it underscores the significance of ML in extracting valuable insights from RWD, detailing various methodologies from classification to dimensionality reduction. Overall, this paper illuminates the transformative potential of RWD and ML in advancing bioinformatics and shaping the future of healthcare.