Comparison of neurosymbolic programming frameworks in human activity recognition
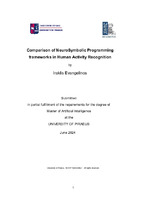
Master Thesis
Author
Evangelinos, Iraklis
Ευαγγελινός, Ηρακλής
Date
2024-06View/ Open
Keywords
Artificial intelligence ; Deep learning ; Neurosymbolic AI ; Complex event recognition ; Human activity recognitionAbstract
Human activity recognition (HAR) is a fundamental task in artificial intelligence, with applications in healthcare, smart homes, and surveillance. Traditional deep learning approaches have achieved state-of-the-art performance in HAR tasks, but they often lack interpretability and struggle to capture complex semantic relationships between activities. Neurosymbolic AI, which combines the strengths
of symbolic reasoning and deep learning, offers a promising alternative.
This thesis explores the application of NeuroSymbolic AI to HAR using DeepProbLog, a popular neurosymbolic framework that integrates logic-based probabilistic modeling with deep learning. We focus on DeepProbLog as it is more extensively used in the field and has shown great promise in various applications. Our primary goal is to investigate how well DeepProbLog performs in recognizing human activities and compare its results with traditional deep learning approaches.
We first introduce the DeepProbLog framework and discuss its underlying principles, including the integration of logic-based probabilistic modeling with neural networks. We then present a comprehensive evaluation of DeepProbLog's performance in HAR tasks, highlighting its strengths and limitations. Our experiments demonstrate that DeepProbLog outperforms traditional deep learning methods in terms of accuracy and interpretability, particularly when dealing with complex activity relationships.