Μεθοδολογίες βαθιάς μάθησης για την πρόβλεψη μεταναστευτικών δικτύων
Deep learning methodologies for migration network prediction
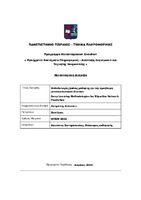
View/ Open
Keywords
Graph convolutional neural networksAbstract
From the earliest times, humanity was on the move. Some people were leaving their homes in
search of work, economic opportunities or to study. Others moved to escape conflict, persecution
or war. Instead, some people migrate as they face the effects of climate change, natural disasters
or other environmental factors. Understanding global migration patterns has important
implications for economic policies, humanitarian assistance and social dynamics. However,
forecasting these migration flows is inherently complex due to the multifaceted interaction of
economic, political and environmental factors.
The purpose of this thesis aims to develop a sophisticated forecasting model using Graph
Neural Networks (GNN) to analyze and predict future migration linkages. By considering
migration as a dynamic network, the model seeks to reveal hidden patterns and possible future
trends in global human mobility.
The methods to be used consist of deep learning algorithms. First, we present the basic
theory on which this thesis is based. Then, we process our data in such a way that we create a
network for each time period. Having these graphs, we analyze the graphs with a focus on the
first and last period 1990-2020. In addition, we create the models to predict future
links(connections). Finally, we conclude the paper by examining the accuracy of each model and
present new methods and the future of the field of deep machine learning on graphs.