Causal and contextual information extraction and data enrichment methods with emphasis on artificial intelligence-driven optimization algorithms for cloud and edge computing
Μέθοδοι ενίσχυσης συνόλων δεδομένων βάσει εξαγωγής αιτιακών χαρακτηριστικών και χαρακτηριστικών πλαισίου αναφοράς με έμφαση σε αλγόριθμους τεχνητής νοημοσύνης για τη βελτιστοποίηση υπολογιστικών υποδομών cloud και edge
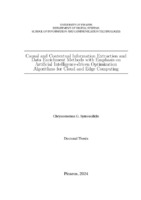
Doctoral Thesis
Author
Symvoulidis, Chrysostomos G.
Συμβουλίδης, Χρυσόστομος
Date
2024View/ Open
Keywords
ανακάλυψη αιτιότητας ; επίγνωση πλαισίου αναφοράς ; δημιουργία αιτιακών χαρακτηριστικών ; αναγνώριση επιρροής ; υπολογιστικά νέφη ; υπολογιστική επεξεργασία στη περιφέρεια, ; μηχανική μάθηση ; βαθειά μάθησηAbstract
Causal Discovery and Context Awareness are central subjects in research for many years. With its origins deeply rooted in scientific research, statistical inference, and philosophical questions, causality has thrilled researchers due to its key role in revealing the relationships between variables. Its significance lies in the pursuit of understanding of not just what happens, but why it happens, and thus making it vital in many scientific fields. Context awareness on the other hand, refers to the ability of a system to understand the context and what are the factors that affect the environment in which it is being used. That being said, Causal Discovery and Context Awareness are of crucial importance when it comes to the development of Artificial Intelligence systems that can be more accurate, robust and generalizable. This thesis focuses on the design and implementation of two Contextual and Causal Extraction methods which can be used for the enrichment of datasets towards the improvement of Machine Learning models. The first method identifies the most influential instances in a dataset and utilizes them in order to generate an Influence-based dataset. The second method discovers the causal relationships that may exist in a dataset and afterwards utilizes this information to generate causal features in order to incorporate this information to the initial dataset. In order to evaluate the methods' performance they have been applied in several, diverse frameworks and strategies used for the optimization of Cloud and Edge Computing environments. The results show that the methods can effectively extract contextual and causal information from datasets. Furthermore, the evaluation proves that the utilization of this information can significantly improve the performance of Machine Learning models. This thesis also provides potential directions for future research that could build upon the findings of the current thesis.