In the heart of data : machine learning applications for improved heart failure outcome prediction
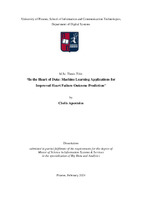
Master Thesis
Author
Chelis, Apostolos
Χέλης, Απόστολος
Date
2024-02View/ Open
Keywords
Machine learning models ; Data mining ; Performance metrics ; Hyperparameter tunning ; Biomedical informatics ; Oversampling ; Undersampling ; Feature selection ; Principal component analysis ; Binary classification ; Μοντέλα μηχανικής μάθησης ; Εξόρυξη δεδομένων ; Μετρικές απόδοσης ; Βελτιστοποίηση υπερπαραμέτρων ; Βιοϊατρική πληροφορική ; Υπερδειγματοληψία ; Υποδειγματοληψία ; Επιλογή χαρακτηριστικών ; Ανάλυση κύριων συνιστωσών ; Δυαδική κατηγοριοποίησηAbstract
The aim of this dissertation is to investigate and evaluate the effectiveness of
various machine learning models (Random Forest, Decision Tree, Support Vector
Machine, Logistic Regression, k-Nearest Neighbors and Gradient Boosting) and
methods (Feature selection, Undersamlig, Oversampling and Principal component
analysis), in predicting the occurrence of death events in patients with heart failure. The
study employs a diverse set of models on a dataset comprising medical records of heart
failure patients, released by Ahmad et al. (2017). Through rigorous analysis,
hyperparameter optimization, and exploration of data preprocessing techniques, the
research seeks to develop a robust framework capable of accurately classifying heart
failure patients based on their risk of experiencing a death event. The findings aim to
contribute valuable insights to the evolving landscape of precision healthcare.
Specifically, by testing and subsequently selecting the most effective methods/models
of machine learning and addressing critical factors/errors present in the datasets, a more
efficient determination of results is achieved.