Non-coding RNA classifier
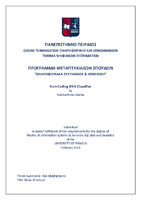
Master Thesis
Συγγραφέας
Vasilas, Konstantinos
Βασιλάς, Κωνσταντίνος
Ημερομηνία
2024-02Επιβλέπων
Maglogiannis, IliasΜαγκλογιάννης, Ηλίας
Προβολή/ Άνοιγμα
Λέξεις κλειδιά
Non-coding ; RNA ; AI ; ClassificationΠερίληψη
This thesis presents a simplified yet effective deep learning model for the classification of non-coding RNAs (ncRNAs). Non-coding RNAs play a vital role in gene regulation and are associated with various biological processes and diseases. The complexity and diversity of ncRNAs make their classification a challenging task. To tackle this, a new neural network model called NCC was developer specifically designed to recognize patterns in ncRNA sequences as well as an updated collection of non-coding RNA sequences datasets to train and test the proposed architecture. The NCC model’s performance, when bench marked against traditional classifiers and existing RNA tools, revealed a 6% improvement in accuracy over the previously best-performing models, reaching 92.69% accuracy, along with slight enhancements in reliability, while still retaining its uncomplicated architecture. This model was trained and evaluated using a newly developed dataset that is ten times larger than the conventional dataset, achieving an accuracy rate exceeding 98%. The model’s accuracy and interpretability hold potential for future research in genomic analysis and the identification of novel ncRNAs.