Investigation of machine learning-based schemes for the development of coarse-grained force fields for organic molecules
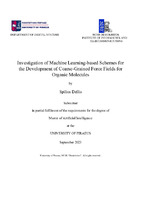
Master Thesis
Author
Dellis, Spilios
Δελλής, Σπήλιος
Date
2023-09View/ Open
Keywords
Molecular dynamics simulation ; Graph Convolutional Neural Network ; SchNet ; Multi-component loss function ; Multi-objective optimizationAbstract
This master thesis presents the development of an innovative evaluation and ranking protocol that integrates physical insights to assess the performance of models and employs statistical
tests for validation. The protocol was applied to address a multi-objective optimization problem
related to self-adapting the weights of a Graph Convolutional Neural Network model. Specifically, the Graph Convolutional Neural Network model was designed to simulate a force field for
predicting molecular system configurations in a coarse-grained setting. The SchNet architecture, a deep learning framework tailored for atomistic systems and capable of modelling quantum interactions in molecules, served as the foundation for the proposed approach. The training
dataset consisted of multiple frames obtained from atomistic simulations of benzene molecules.
This research mainly focused on achieving a self-balancing of weights within the dual components of the Graph Convolutional Neural Network model’s loss function. This self-balancing
aimed to optimize the trade-off between different objectives in the multi-objective optimization
problem, enhancing the model’s performance. To identify the most effective self-balancing
method among various alternatives, the developed protocol was employed to evaluate the performance of each approach. The evaluation results revealed the most optimal self-balancing
approach for this specific multi-objective optimization problem within the context of simulating a force field for predicting coarse-grained molecular system configurations. The presented
methodology offers a valuable contribution to the field of deep learning applied to atomistic
systems and multi-objective optimization, paving the way for further advancements in molecular dynamics simulations and related research.