Detecting Alzheimer’s disease using NLP methods
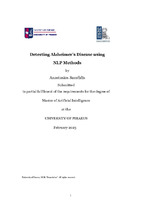
Master Thesis
Author
Σαραφίδης, Αναστάσιος
Sarafidis, Anastasios
Date
2023-02View/ Open
Keywords
Machine learning ; ADReSS ; Feature engineering ; Alzheimer's disease detection ; Natural language processingAbstract
Alzheimer's disease (AD) is a progressive brain disease that cannot be treated, but only be slowed down or stopped with medical treatment. Language changes may indicate that a patient's cognitive functions have been compromised, potentially
leading to an earlier diagnosis. The challenging manual diagnosis of such neurodegenerative disorders could be assisted by the use of Machine Learning algorithms able to automatically detect those disorders using linguistic features. For this purpose, we use the ADReSS Challenge dataset and we develop NLP methods to classify and analyze the linguistic characteristics of Alzheimer's disease patients. To distinguish between language samples from probable AD and control patients, we propose the implementation of an XGBoost classification model, which has not been present in similar cases literature, along with three other models that are most often used. XGBoost’s final scores indicate that this classification model, with the right adjustments in terms of data and features, is able to compete with or even surpass in performance the other models.