Μοντελοποίηση και βραχυπρόθεσμη πρόβλεψη των τιμών χρονοσειράς κρυπτονομισμάτων, χρησιμοποιώντας τεχνικές μηχανικής μάθησης
Modeling and short term forecasting of cryptocurrency time series prices using machine learning techniques
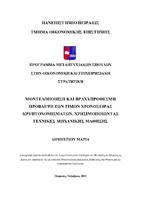
View/ Open
Keywords
Κρυπτονομίσματα ; Μοντέλα πρόβλεψης ; Ανάλυση χρονοσειράς ; Μηχανική μάθησηAbstract
Due to their growing popularity and commercial acceptance, cryptocurrencies are playing an increasingly essential role in changing the financial system. While many people invest in cryptocurrency, the dynamic characteristics and predictability of them are still largely unknown, putting investments at risk. Machine learning is called upon to tackle the problem of predicting these currencies. Many researches are trying to identify the best model for predicting the price of Bitcoin, as the strongest one, leaving a significant research gap in the prediction of other cryptocurrencies as well. A comprehensive investigation will identify the best possible model with remarkable results in the forecast field.
In this paper, we attempt to predict the price of four cryptocurrencies (Bitcoin, Ethereum, Ripple and Tether) taking into account a variety of factors that affect their value with the highest possible accuracy. Three models of different logic were chosen as prediction methods. With the help of Python and its libraries, the traditional Autoregressive Integrated Moving Average (ARIMA) model, the Long Short-Term Memory model (LSTM) and the decision tree structure model Random Forest (RF) were applied to the time series data. The predictions were tested on the real time series data and the root mean square error (RMSE) was applied to evaluate and compare the models.
"Can researchers and investors' dream of cryptocurrency price prediction become a realistic scenario?". While the traditional Arima method and the RF decision tree method did not provide satisfactory predictions, the LSTM model showed promising results. The LSTM model can retain prior information, which can greatly help improve the model's ability to learn signal sequences and inherent non-linear patterns.