Query optimization with deep learning architectures
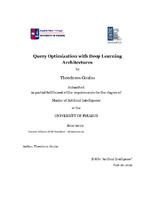
Master Thesis
Author
Goulas, Theodoros
Date
2022-06View/ Open
Abstract
The increasing trend of moving from the old-fashioned centralized database systems into distributed ones significantly increased the query optimization problem's complexity, leading to complicated optimization algorithms based on time and resource-consuming analytical methods.
This study proposes introducing natural language processing techniques combined with Deep Learning architectures as a statistical alternative to the traditional analytical query optimization approach to address this issue.
In the context of this paper, based on the assumption that both the queries in a database and the corresponding optimal execution plans are text sequences, it was investigated whether a sequence-to-sequence deep neural network (Neural Machine Translation) architecture can adequately predict or approximate the optimal execution plan, given a query in an existing database.
The experiment was based on the CoSQL dataset which was loaded into a PostgreSQL database and used to generate the experimental dataset. Using the EXPLAIN command for each query on the database, the corresponding optimal execution plan was generated by the database optimizer. The text sequence of each query was fed as input to the neural network and the optimal execution plan was used as the output for model training.
The conducted experiments indicated that the complexity and the sparsity of the input and output sequences exceed the learning capabilities of the proposed deep neural network, producing inefficient or even non-trainable (resource-wise) models. However, the examined architecture showed promising results in extracting valuable insights that the ordinary optimizers can use as hints to conclude faster and more accurate decisions during the optimization process regarding operators' implementation and execution order.