Evaluation of machine learning models for predicting the system marginal price of an electricity system – Italian SMP Day-Ahead forecasting
Αξιολόγηση μοντέλων μηχανικής μάθησης για πρόβλεψη της οριακής τιμής συστήματος ενός συστήματος ηλεκτρικής ενέργειας – Πρόβλεψη της Προ-Ημερήσιας Ιταλικής ΟΤΣ
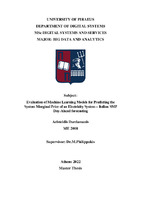
View/ Open
Keywords
Machine learning ; RNN ; LSTM ; MLP ; SARIMAX ; Autoregression ; SMP ; Forecasting ; Day-AheadAbstract
In a power market, electricity price forecasts are of great importance for market participants. The presence of nonlinearity, nonstationarity, and multiple seasonality in electricity prices renders price prediction a challenging task. Many studies have been conducted in this field, however more robust and up to date price forecast methods are required. Europe is a great and complex energy market comprised of many countries who exchange vast amounts of electricity on a daily basis. Italy is one of the most significant energy markets in the southern European area. In this research, three types of machine learning models were investigated with the aim of predicting as much accurately as possible the Italian SMP. Namely, one autoregressive, one MLP and three LSTM models were constructed in order to forecast the Italian Day-Ahead SMP at an hourly level. The predictions of each model were evaluated for a timeframe extending up to two weeks ahead through the calculation of the respective MAPE and RMSE metrices. A number of attributes were initially selected as candidates to train our machine learning models, which is also the key difference between other similar studies. Specifically, among the attributes initially selected, electricity prices of major European countries were included. This is crucial owe to the fact that Italy is now coupled with many neighbouring countries suggesting a much stronger interdependence with its borders. Three feature selection methods were applied to filter out any possible redundant attributes, the SFS, the Random Forest Regressor and the F_regression methods. The Hungarian SMP, the 24 lag SMP price (Italian SMP shifted by 24 hours in the past) and the Residual attributes were determined as the most important. The dataset used covers in total the period of 2021/06/01 to 2021/10/22. Historical data were collected from various sources such as, GME, Nord Pool, Entso-e and Terna. The training and test samples were identical for each model in order to secure consistency. The evaluation results are promising especially for the Bidirectional LSTM and the Stacked LSTM. Namely, concerning a forecast of up to two weeks ahead MAPE and RMSE values of the Stacked LSTM and the Bidirectional LSTM were found to be 6.965 per cent, 19.628 Euro / MWh and 7.249 per cent, 20.237 Euro / MWh respectively. In addition, these proposed deep learning algorithms showed an improved performance when trained with a bigger sample of data. However, there are more risks adding to the forecast error, which originate from the uncertainty in the prediction of both the Hungarian SMP and the Residual features. On the grounds that Stacked LSTM produced the most prominent results, a sensitivity analysis was performed on it to check at what extent the model’s performance would change. It was decided to alter by +15 per cent and by -15 per cent the values of HU. The performance of the model deteriorated and more specifically for a +15 per cent change MAPE and RMSE increased to 8.720 per cent and 23.648 Euro /MWh correspondingly, while for a -15 per cent change MAPE and RMSE increased to 8.856 per cent and 24.561 Euro / MWh respectively.