Αναλυτική δεδομένων αγώνων καλαθοσφαίρισης για την πρόβλεψη αποτελεσμάτων και εξαγωγή γνώσης
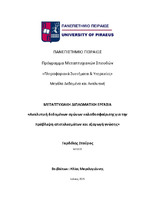
View/ Open
Keywords
Μηχανική μάθηση ; Aναλυτική αθλητικών δεδομένων ; Κατηγοριοποίηση ; Πρόβλεψη μπάσκετ ; Euroleague ; NBA ; Multi-layer perceptron ; k-nearest neighbors ; Logistic regression ; Support Vector Machines (SVM) ; Random forest ; Pearson correlation ; Machine learning ; Classification ; Sports analyticsAbstract
The present thesis deals with Sports Analytics. As in many other domains, also in sports the rate of data collection has been steadily increasing in recent years. Basketball Data were used.
More specifically, two datasets from the two largest leagues in the world, the NBA and the Euroleague, were analyzed. Each dataset contains statistics for the seasons 2005-06 up to 2018-19. Apart from the many statistics that concern data of a match such as points, assists, rebounds, steals, etc. is given the success of admission or not in playoffs of the respective league. Attribute that we will deal with, since it is the class of each dataset.
The following Supervised Machine Learning algorithms were used to present a model that can make more efficient predictions per league. The algorithms are Logistic Regression, k-nearest neighbors (KNN), Support Vector Machine (SVM), Random Forest, Neural Network (Multi-layer Perceptron). They were used in 3 different scenarios involving processed data with different techniques such as standardization, Pearson correlation and average statistics.
Another goal was to make a forecast of the last 5 years with the above classifiers, based on previous years for each dataset.
As the last part of this thesis was to use the best model/classifier per league/dataset and fit it to the opposite league.