Big Mobility Data Analytics: algorithms and techniques for efficient trajectory clustering
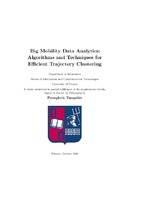
Doctoral Thesis
Author
Tampakis, Panagiotis
Ταμπάκης, Παναγιώτης
Date
2019-10View/ Open
Keywords
Mobility data mining ; Sub-trajectory clustering ; Trajectory segmentation ; Trajectory sampling ; MOD engines ; Cluster analysis ; Temporal-constrained (sub-)trajectory clustering ; Moving objects ; Indexing ; (Sub)Trajectory Join ; Distributed Join Processing ; MapReduce ; Mobility data ; Subtrajectory clustering ; Big mobility data mining ; Distributed clustering ; Trajectories ; Big dataAbstract
The unprecedented rate of trajectory data generation that has been observed during the recent years, caused by the proliferation of GPS-enabled devices, poses new challenges in terms of storage, querying, analytics and knowledge extraction from mobility data.
One of these challenges is cluster analysis, which aims at identifying clusters of moving objects according to the similarity degree of their movement. Discovering clusters of moving objects is an important operation when trying to extract knowledge out of mobility data, since by doing so, the underlying hidden patterns of collective behavior can be unveiled. What is even more challenging is treating knowledge discovery techniques, such as cluster analysis, as an integral part of a real DMBS, which can turn out to be practical and useful in real-world application scenarios, where issues like the ease of use (e.g., via a simple SQL interface) are taken into consideration. Furthermore, the support of incremental and progressive cluster analysis in the context of dynamic applications is of great interest, where (i) new trajectories arrive at frequent rates, and (ii) the analysis is performed over different portions of the dataset, and this might be repeated several times per analysis task. However, performing such “expensive” operations over immense volumes of data in a centralized way is far from straightforward, which in turn calls for parallel and distributed algorithms to address the scalability requirements posed by the Big Data era. The bottleneck of performing “expensive” operations, such as cluster analysis, is the underlying join query. Joining trajectory datasets is not only the cornerstone of various trajectory cluster analysis methods, but it is also a significant operation in mobility data analytics with a wide range of applications, such as carpooling, suspicious movement discovery, etc. In this thesis, we aim to address the above challenges.
Towards this direction, we propose a novel in-DBMS Sampling-based Sub Trajectory Clustering algorithm, namely S2T-Clustering, which is incorporated in a real MOD engine over an extensible DBMS (PostgreSQL in effectively than state-of-the-art techniques. Moreover, we introduce the temporally-constrained subtrajectory cluster analysis problem. To address it, we propose ReTraTree, an indexing scheme which organizes trajectories by using an effective spatio-temporal partitioning technique. Partitions in ReTraTree correspond to groupings of subtrajectories, which are incrementally maintained and represented via a hierarchical organization of a small (thus, light-weight in-memory) set of ‘representative’ subtrajectories. Given this, the problem in hand can be efficiently solved as a query operator on ReTraTree, coined QuT-Clustering. Our approach further contributes to the mobility data management and mining domain for the additional reason that it has been designed and implemented in a MOD engine. Such functionality enables the application users to perform progressive cluster analysis via simple SQL in a real extensible DBMS. Furthermore, we propose an efficient in-DBMS architecture for progressive time-aware subtrajectory cluster analysis, by utilizing the aforementioned in-DBMS solutions along with a Visual Analytics (VA) tool to facilitate real world analysis.
Towards addressing the challenges posed by the Big Data era, we introduce the Distributed Subtrajectory Join query, an important operation in the spatiotemporal data management domain, where very large datasets of moving object trajectories are processed for analytic purposes. To address this problem in a scalable manner, we follow the MapReduce programming model. Finally, we address the problem of Distributed Subtrajectory Clustering by building upon the Distributed Subtrajectory Join query, in order to tackle the problem in an efficient manner. We propose two alternative trajectory segmentation algorithms and a distributed clustering algorithm where the clusters are identified in a parallel manner.