Πρόβλεψη χρονοσειρών αέριας ρύπανσης και ενεργειακής κατανάλωσης με τη χρήση ARIMA και LSTM μοντέλων
Time series prediction of pollution and energy consumption data using ARIMA and LSTM models
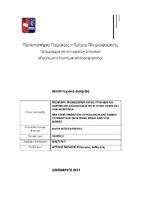
View/ Open
Keywords
ARIMA models ; LSTM ; Χρονοσειρές ; Κατανάλωση ενέργειας ; Αέριοι ρύποιAbstract
There has been great development in the field of Machine Learning in recent years, mainly since the processing power of the machines that make use of its capabilities has greatly increased. This has sparked the interest of developers and analysts who are trying and have managed to develop different models based on machine learning techniques that apply to a wide range of applications.
This thesis deals with a large chapter of machine learning science, that of neural networks and in particular the LSTM recurrent neural networks. In addition, by using timeseries analysis methods with the development of ARIMA models, we test their performance by comparing each one of them. Three different datasets were used for this study and the model’s development. The first concerns the prediction of the energy consumption by a household, the second concerns the air quality forecasting for a particular area, and the third also concerns the prediction of air quality but for many natural sites. Their use required the data preparation by managing NaN values and by separating them into training datasets and tests datasets. Several variants of the ARIMA and LSTM basic models were tested, and we reached to the best of them, while the measurements used for performance were RMSE and MAE metrics.
This analysis shows the capability of both recurrent neural networks and timeseries analysis methods on solving big and complex problems like the examined.