Θεματική μοντελοποίηση σε σώμα ειδησεογραφικών κειμένων
Topic modelling in a news headline corpus
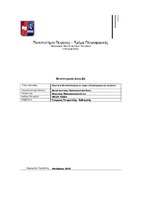
View/ Open
Keywords
Topic modelling ; Topic coherence ; Topic over time ; LDA ; Θεματική μοντελοποίησηAbstract
News articles is a huge structure of historical documents. They are a valuable resource to study the past. Native Language Processing is a field of Artificial Intelligence that helps computers understand, interpret and manipulate human language. The task of understanding natural language is to be able to extract meaning from words, sentences, paragraphs, and documents. At the document level, one of the most useful ways to understand the text is to analyze its subjects - topics. Topic Modeling is an important tool in analyzing historical documents. Topic Modeling provides a way to analyze a large volume of unclassified text. A topic contains a set of words that often appear together. We have an unsupervised learning problem to be implemented. In unsupervised learning, the system needs only to discover associations or groups in a set of data, creating patterns, without knowing anything about this. Historical documents are often complicated, difficult to categorize and may not have standard spelling and formatting. In this work from a corpus of news headlines from 2003 to 2017, of ABC News, we are trying to implement standard pattern works that we can get all the deep learning information. In our case, we only have unlabelled input data and we need to define endogenously the categories of topics. The modeling of topics is quite similar to a Clustering problem.
In this work, we compare the algorithms that implement Topic Modeling, examine why Topic coherency do not work in our case and implement Topic Modeling in a corpus of documents from news headlines. We analyze the Topics, and we find the dominant Topic per document and its evolution over time. Then we present the evolution of Topics over time and finally we add new headlines and how our algorithm classifies them in the most appropriate topic.