Κατανεμημένη ενισχυτική μάθηση για έλεγχο και διαχείριση ενέργειας απομακρυσμένων αυτόνομων φωτοβολταϊκών μικροδικτύων
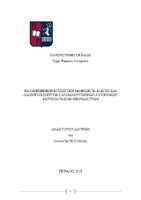
View/ Open
Abstract
This PhD dissertation proposes solutions in order to address the problem of energy management in islanded photovoltaic microgrids. In the islanded mode, the main issue that needs to be addressed, is the provision of electricity in order to maintain, if not increase, the reliability of the microgrid by the uncertainties which are introduced by the renewable energy sources and by the stochastic demand of the consumers. In this PhD dissertation, a reinforcement learning agent is proposed to solve the problem of energy management in an islanded photovoltaic microgrid consisting of a photovoltaic source, a battery, a dynamic load and a desalination unit. The agent is based on reinforcing learning and has the ability to learn strategies by interacting with the environment. The agent does not only manage the battery but also manages the desalination plant which satisfies the demand of the potable water. The simulations results present good performance in energy management but in some cases the energy demand could not be covered or the battery was subjected to many deep discharges. These drawbacks are generally caused by three reasons. The first reason is the general discretization of the state space in combination with the simple exploration/exploitation algorithm of the agent (results to incomplete exploration of the state space). The second reason, is the simple reward function which causes slow convergence of the algorithm and the third reason is the slow operating cycle of the agent that did not perceive changes in produced and demanded power. Taking into account the aforementioned drawbacks, some modifications are applied to the agent. New state variables are defined and a fine grained discretization is performed, a more sophisticated algorithm for exploration/exploitation is used, a new reward arises by a fuzzy logic system structured by subjective rules and the discrete time step of the agent is reduced. The simulation results indicate the further improvement of the battery management as well as the further improvement regarding the coverage of the energy.
In this PhD dissertation, it is also proposed a multiagent system based on a modified independent learners approach. This multiagent system is designed for the energy management of a more complicated islanded photovoltaic microgrid consisting by several units (photovoltaic unit, desalination unit, electrolytic unit, fuel cell, diesel generator, dynamic load). By this way, the problem is addressed in a fully decentralized framework without the use of a coordinating agent. Agents do not have built-in knowledge and learn through interaction with the environment. This fact allows them to adapt their strategy to any changes that the microgrid may be subjected. The modified approach lies on the fact that it uses only local rewards and/or state information that are relevant to each agent. This has the effect of reducing the state space and strengthening the learning mechanism. In order to cope with the continuous state-action space, instead of simple discretization, the fuzzy Q-learning was introduced to each agent. By this way, the power dispatch is allowed amongst the units as they can operate at partial load conditions.
Furthermore, in order to solve the problem of energy management, it was necessary to develop methods and solve problems directly related to the main problem. In particular, in order to use the desalination unit in the simulations, a model of the process has to be developed. The model has as input the power consumption and as output the water production and the water quality (electrical conductivity). The model is data driven. The data were collected by a reverse osmosis desalination unit, which is installed at the Agricultural University of Athens. ANFIS (Adaptive Neuro-Fuzzy Inference System) was used as a modeling tool in order to develop the model without the use of mathematical equations.
Also, the problem of the Maximum Power Point Tracking (MPPT) of the photovoltaic sources had to be solved. The considered microgrid is a photovoltaic islanded microgrid. It means that there is no connection to the electrical grid and the only renewable source of the microgrid is a photovoltaic source. This makes it imperative to exploit as much energy as possible from the photovoltaic source. The Maximum Power Point (MPP) is a unique point in the voltage-current curve where the power produced by the photovoltaic source is maximized. The operating point of a photovoltaic source is affected by the environmental conditions (temperature and solar irradiance) and by the load which is connected. The objective is the operation point of the photovoltaic source to be coincident with the MPP at any time instant. In addition, another problem that needs to be solved in the photovoltaic sources is the conditions of partial shading. In partial shading conditions, the characteristic current-voltage curve of the photovoltaic source gains multiple local maxima, which makes the tracking of the global MPP very difficult. In this PhD dissertation, two solutions are proposed for the MPPT control problem. In particular, a methodology, which uses a single neuron in order to achieve MPPT, is proposed. The neuron combines online learning, based on the gradient descent method and offline learning, based on the metaheuristic algorithm of the “Big Bang Big Crunch” (BB-BC). The parameters that need optimization are only four, the initial values of the three weights of the neuron and the slope of the sigmoidal function. Additionally, the single neural controller in combination with the cascaded dc/dc converter topology is proposed to solve the problems which are introduced by the partial shading conditions. In this topology, each photovoltaic module has its own controller and its own converter. The maximum power is extracted from each module and the total power is applied on the load via the converters. In addition, a universal photovoltaic maximum power point tracker based on reinforcement learning, is suggested. This approach can achieve MPPT by using only two parameters, the short circuit current and the open circuit voltage of the photovoltaic source at the Standard Test Conditions (STC: 25OC & 1000W / m2). The agent interacts with the environment and learns to act appropriately not only in different environmental conditions but also in variable electrical loads with very small errors and no oscillations around the MPP.